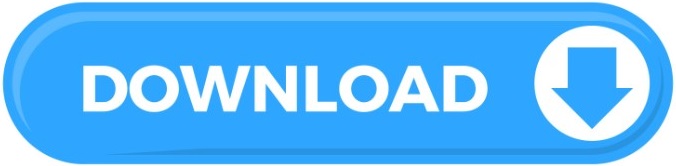
StackGAN and StackGAN++ use multi-scale GANs to scale up the image resolution and improve visual fidelity. The embeddings are produced by an encoder pretrained using a contrastive loss, not unlike CLIP.
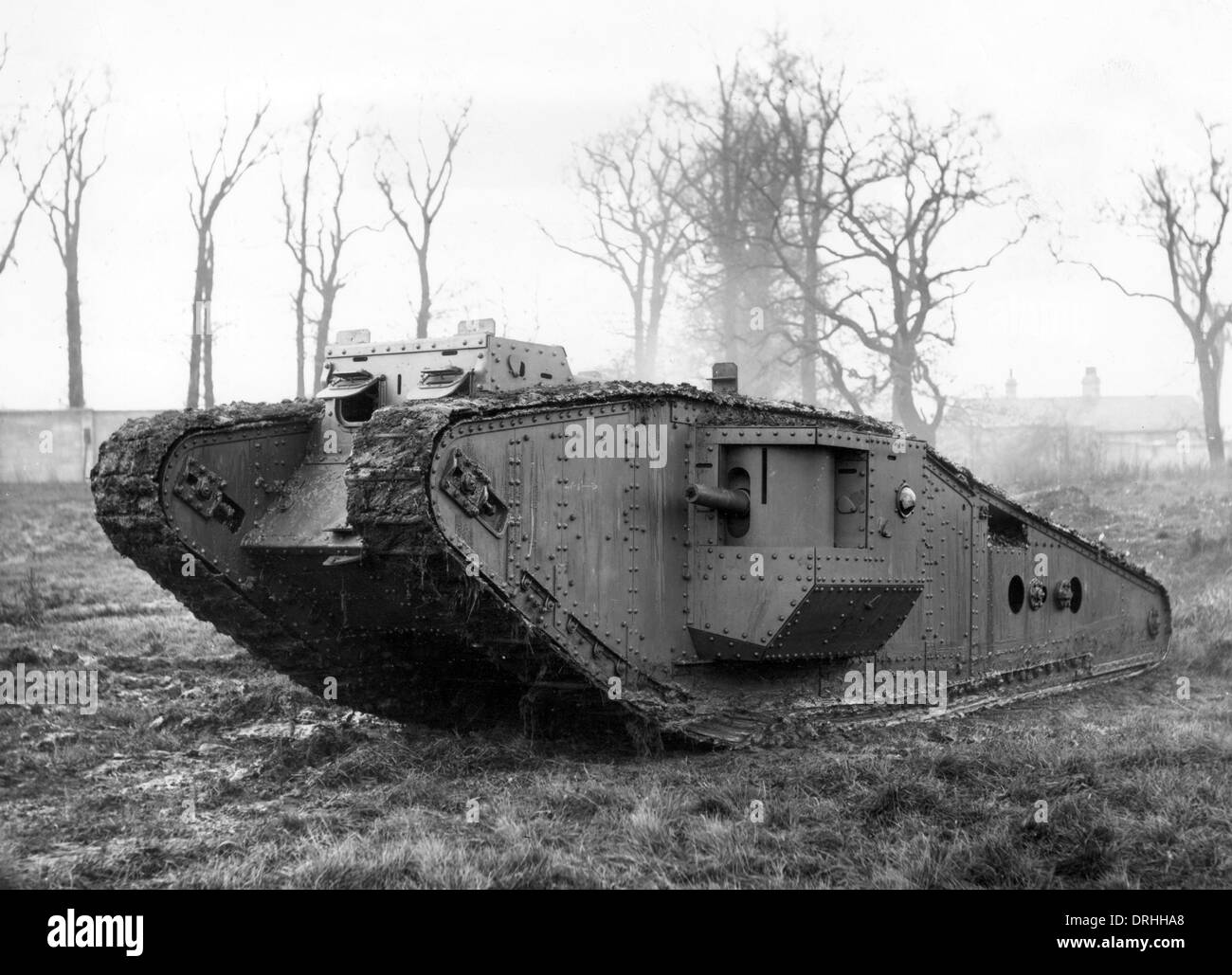
al, whose approach uses a GAN conditioned on text embeddings. Text-to-image synthesis has been an active area of research since the pioneering work of Reed et. We provide more details about the architecture and training procedure in our paper. DALL♾ uses the standard causal mask for the text tokens, and sparse attention for the image tokens with either a row, column, or convolutional attention pattern, depending on the layer. The attention mask at each of its 64 self-attention layers allows each image token to attend to all text tokens. Finally, the transformations “a sketch of the animal” and “a cell phone case with the animal” explore the use of this capability for illustrations and product design.ĭALL♾ is a simple decoder-only transformer that receives both the text and the image as a single stream of 1280 tokens-256 for the text and 1024 for the image-and models all of them autoregressively. Those that only change the color of the animal, such as “animal colored pink,” are less reliable, but show that DALL♾ is sometimes capable of segmenting the animal from the background. Other transformations, such as “animal with sunglasses” and “animal wearing a bow tie,” require placing the accessory on the correct part of the animal’s body. This works less reliably, and for several of the photos, DALL♾ only generates plausible completions in one or two instances. The transformation “animal in extreme close-up view” requires DALL♾ to recognize the breed of the animal in the photo, and render it up close with the appropriate details. The most straightforward ones, such as “photo colored pink” and “photo reflected upside-down,” also tend to be the most reliable, although the photo is often not copied or reflected exactly. We find that DALL♾ is able to apply several kinds of image transformations to photos of animals, with varying degrees of reliability.
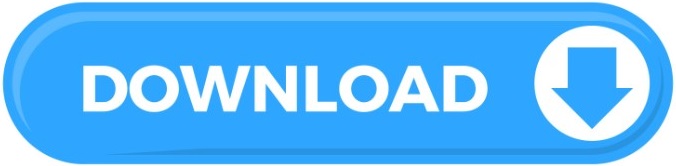